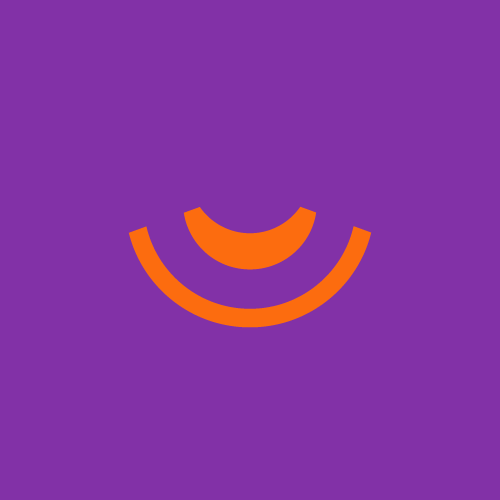
Writen by ilegra, think beyond, in 11/11/2020
3 minutes of reading
Data Engineer: why is this professional so relevant in a Data Science project?
The Data Engineer is focused on building pipelines that provide quality information ready to be analyzed, enabling the intelligent use of data.
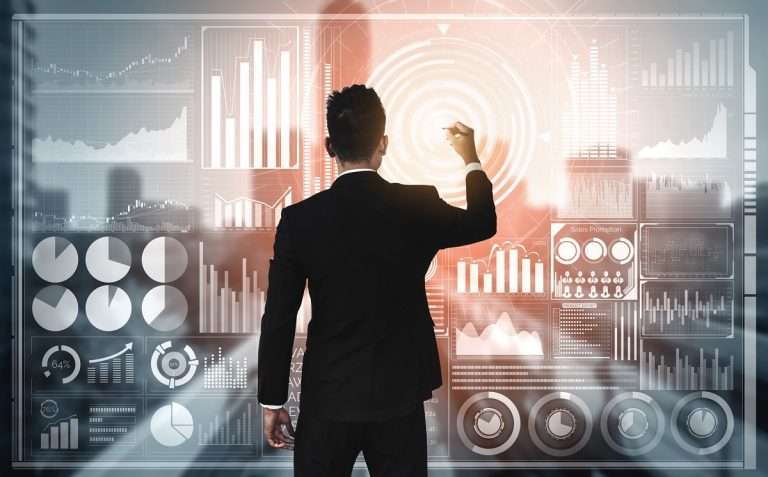
When I say I am a Data Engineer, when I introduce myself to people, normally I have to explain what I do. Often I give up and I say I am a developer, that makes it easier, but it is not entirely true.
However, it shocks me that I have to explain to people in technology what I do, as it is common that they take my profession as data scientist, data architect or even database administrator. It gets even more confusing when I say I am an expert in data processing using cloud computing.
For you not to be disappointed when you start reading this article, I would like to clarify what a data engineer does. Gartner Consulting has a definition that I think is practically perfect about the role of data engineering within the technology world:
“The primary responsibility of data engineers is to build data pipelines that would provision quality data ready for analysis. Building data pipelines often requires multiple iterations. This often involves enrichment and integration of input datasets, which is done in order to build a meaningful data input in support of the model development. It requires a strong focus on data integration, modeling, optimization, quality, governance and security.”
Now that we have a definition from a reliable source, I would like to highlight and explain a little better four points that I consider to be the main ones in this definition:
Build data pipelines
The famous ETL (extract, transform and load) is a clear example of a data pipeline. The difference is that often time we do not need to transform data, only extract them from one place and insert somewhere else. A data pipeline is a set of a flow of operations about the data, which follows a specific order and has defined conditions and decisions. Generally, we have to insert data in a new repository, combining several data from different sources, which takes us to the next highlight.
Enrichment and integration of input datasets
Enriching the data that is being integrated means to improve and/or creating intelligent information within those data sets. Data enrichment may happen through complementary information contained in different sources or even by executing machine learning algorithms, such as clusterization or classification ones to categorize data that are being processed. However, when we cross information from different origins, and also, when we make treatment and transformation on the data, we have to ensure our next topic.
Quality data ready for analysis
I always describe the final repository as a repository, not as a database or any other specific data structure. I do this because we can have one or more endpoints for our data pipeline, and regardless of the endpoint, the information contained in them should be quality information, consistent for the analysis and consumption of the users or applications.
Strong focus on data integration, modeling, optimization, quality, governance and security
This last item I highlighted here in the description of data engineering by Gartner is basically the summary of all operations that are the focus of data engineering. The data integration work can be its core, with the task of building data pipelines as the main task. However, the data engineer needs to have excellent knowledge of data modeling, optimization (of data and software), data quality, data governance, and of the levels of security and access attributed to the data.
Now you know more about the delivery of a data engineer. You can keep reading about trends and data in the article,
Which technology and consumption trends should be considered for the upcoming years?